Introduction:
Machine Learning (ML) has witnessed remarkable advancements and is powering innovations across various industries. However, it's important to acknowledge that ML isn't a silver bullet that can solve every problem. In this blog, we will explore the limitations of machine learning in recent technologies and scenarios where it might fall short despite its immense potential.
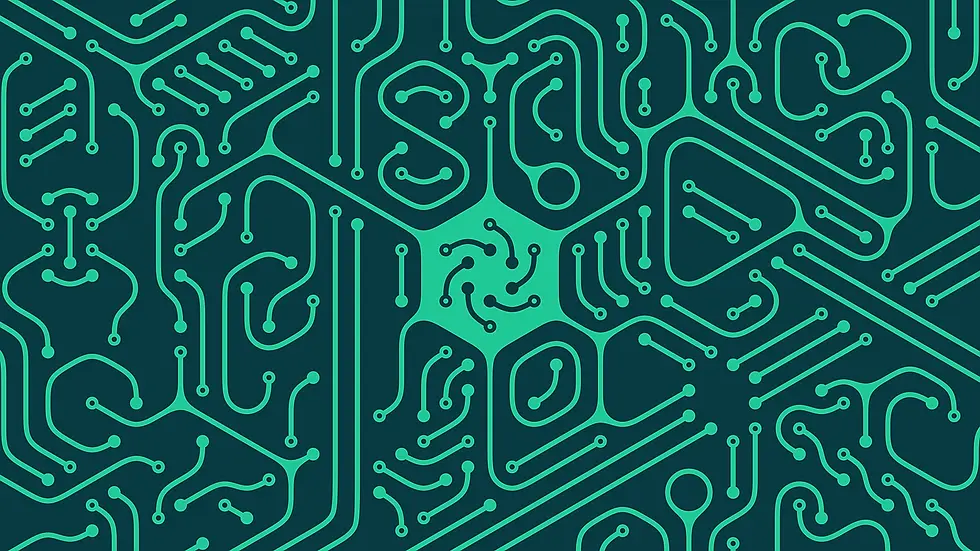
1. Lack of Common Sense and Contextual Understanding:
Machine learning models lack true common sense and an inherent understanding of context. While they excel in pattern recognition, they struggle with grasping the nuances of human experience and reasoning. As a result, ML algorithms might fail in scenarios that require deep contextual understanding, such as irony or sarcasm detection in text.
2. Creativity and Abstract Thinking:
Despite generating impressive content like art, music, and text, machine learning models can't replicate human creativity and abstract thinking. They can mimic styles and trends but can't truly innovate or produce something entirely novel.
3. Ethical and Moral Decision-Making:
Machine learning algorithms are as good as the data they are trained on. They can inadvertently learn biases present in the data, leading to ethical and moral challenges. Decisions involving ethical judgment or complex moral considerations are beyond the capabilities of ML models.
4. Real-Time Learning and Adaptation:
While ML models can learn from historical data, they often lack the capacity for real-time learning and dynamic adaptation to rapidly changing situations. In scenarios where immediate adaptation is critical, such as emergency response systems, ML might not be optimal.
5. High-Level Reasoning and Critical Thinking:
Machine learning models struggle with high-level reasoning, abstract thinking, and the ability to critically analyze complex situations. While they can process vast amounts of data, they may not interpret it comprehensively, making them unsuitable for tasks requiring deep analysis.
6. Unstructured and Noisy Data:
While ML excels with structured data, handling unstructured and noisy data remains a challenge. ML models might misinterpret data with inherent noise, leading to inaccurate predictions or decisions.
7. Emotional and Empathetic Intelligence:
Understanding human emotions and exhibiting empathy are areas where ML falls short. While sentiment analysis can identify emotions to some extent, machines cannot truly comprehend emotions like humans can.
8. Domain Expertise and Common Sense Reasoning:
ML models, particularly those with limited training data, may struggle to comprehend domain-specific knowledge and apply common sense reasoning in intricate situations, leading to errors in critical tasks.
Conclusion on What Machine Learning is NOT good at:
Machine learning is a transformative technology, but it's essential to recognize its limitations. It excels in data-driven tasks, yet it's not a substitute for human creativity, ethical judgment, abstract thinking, and domain expertise. As we embrace machine learning in various sectors, it's crucial to understand its boundaries and apply it judiciously, supplementing it with human expertise where needed. By acknowledging these limitations, we can harness the true power of machine learning while maintaining a balanced perspective on its capabilities.
*************** Do know What Machine Learning is NOT good at ******************
Comentários