Retrieval-augmented generation is a natural language processing (NLP) technique that combines elements of both information retrieval and natural language generation to improve the quality and relevance of generated text. It's often used in tasks like content generation, question answering, and text summarisation
Here's how it works:
Retrieval Phase: In the retrieval phase, the system searches a large dataset or knowledge base to find relevant information related to the input or the context. This information retrieval step typically uses traditional search techniques, such as keyword matching or more advanced methods like semantic search.
Augmentation: The retrieved information is then used to augment the generation process. Instead of generating text entirely from scratch, the model incorporates the retrieved content to provide more contextually relevant and accurate responses.
Generation Phase: With the retrieved information as context, the system generates the final output, whether it's a response to a user query, a content recommendation, or a summary of a longer text.
Retrieval-augmented generation has several advantages:
Improved Relevance: By incorporating information from a knowledge base, the generated text is often more accurate and relevant to the user's query or context.
Fact-Based Responses: It helps ensure that the generated content is based on accurate, factual information from the knowledge base.
Reduction in Ambiguity: The retrieved information can help disambiguate user queries or context, leading to more precise answers.
Expanded Knowledge: It allows the system to provide information that it might not have stored in its training data but can retrieve from external sources.
This approach is commonly used in chatbots, question-answering systems, and content generation systems to provide users with high-quality, fact-based responses. It leverages both the generative capabilities of language models and the factual knowledge stored in external sources, striking a balance between creativity and accuracy in text generation.
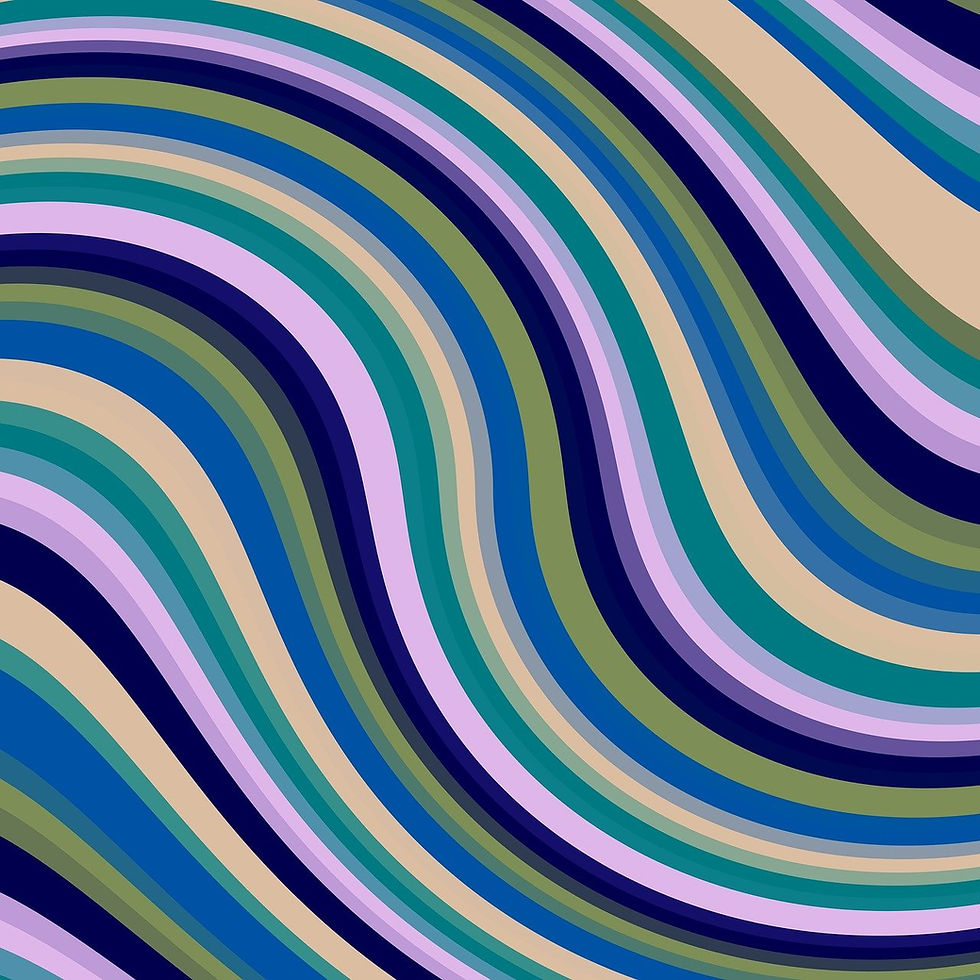
Examples and Usecases of Retrieval-Augmented Generation(RAG)
1. Chatbots and Virtual Assistants:
Example: Consider a customer support chatbot for an e-commerce website. When a customer asks about product specifications, the chatbot can retrieve information from the product database and generate detailed, accurate responses.
Use Case: Retrieval-augmented generation enhances the accuracy and relevance of responses in chatbots, leading to better customer support experiences.
2. Question Answering Systems:
Example: In a medical question-answering system, a user asks about the symptoms of a rare disease. The system can retrieve information from medical literature databases and generate a comprehensive, evidence-based answer.
Use Case: This approach ensures that answers to user queries are based on the latest medical research and are more reliable.
3. Content Generation:
Example: In automated content generation, a news article generation system can retrieve up-to-date statistics and facts from various sources to augment the writing process, producing data-driven and informative articles.
Use Case: This method results in content that is both engaging and factually accurate, making it suitable for news reporting and data-driven content creation.
4. Text Summarization:
Example: A text summarization system that extracts key points from lengthy research papers to generate concise summaries. The retrieval phase identifies relevant sections, and the generation phase condenses the content into a summary.
Use Case: Researchers and professionals can quickly grasp the main findings of extensive documents, saving time and improving information accessibility.
5. Language Translation:
Example: In machine translation, retrieval-augmented generation can improve the translation quality by retrieving contextually relevant phrases or idiomatic expressions from bilingual corpora.
Use Case: This approach results in translations that better capture the nuances and idioms of the target language.
6. Legal Research and Document Generation:
Example: In the legal domain, a system can retrieve relevant case law and statutes to assist lawyers in preparing legal documents such as contracts or briefs.
Use Case: Retrieval-augmented generation helps ensure that legal documents are compliant with current laws and regulations.
7. Content Recommendation:
Example: In recommendation systems, when a user expresses interest in a specific topic, the system can retrieve and generate personalized content recommendations, such as articles, videos, or products.
Use Case: This approach enhances the personalisation and relevance of content recommendations, leading to increased user engagement.
These use cases demonstrate how retrieval-augmented generation enhances various applications by combining the advantages of information retrieval with the creative and context-aware capabilities of natural language generation. It ensures that the generated content is factually accurate, contextually relevant, and highly informative, resulting in improved user experiences and more effective automation of information-related tasks.
In conclusion, Retrieval-Augmented Generation(RAG) represents a pivotal advancement in natural language processing, seamlessly integrating information retrieval with text generation to produce contextually relevant and factually accurate content. This innovative approach has found application in a wide array of domains, ranging from chatbots and virtual assistants to question answering, content generation, and language translation.
By harnessing the power of retrieval-augmented generation, these systems can provide users with highly accurate and reliable information. This leads to more effective customer support, improved user experiences, and the automation of complex tasks that demand both precision and creativity.
Whether it's enhancing content generation, simplifying research, or personalising recommendations, retrieval-augmented generation fosters a harmonious synergy between data-driven facts and creative language generation. The result is a transformation in the way we interact with machines, access information, and engage with content.
As technology continues to advance, retrieval-augmented generation will undoubtedly play a pivotal role in shaping the future of artificial intelligence and human-computer interaction. It offers the promise of more accurate, more personalised, and more engaging interactions with machines, ultimately benefiting businesses and individuals across a broad spectrum of industries.
Dr. Pinnacle can help you implement ML Solutions. We have a deep understanding of ML Observability and the challenges that organisations face in implementing it. We have a proven track record of success in helping organisations implement ML Solutions.
Contact us(info@drpinnacle.com) today to learn more about how we can help you implement ML Solutions.
Comentarios