In recent years, artificial intelligence (AI) has emerged as a powerful tool capable of transforming various industries. From healthcare to finance, AI’s ability to analyze vast amounts of data and make predictions has revolutionized the way we approach problems. However, as with any technology, AI is only as good as the data it is trained on. A growing concern in the AI community is the impact of feeding AI systems with low-quality, biased, or inaccurate data, which can lead to misleading or nonsensical outputs.
The Importance of High-Quality Data
At the core of every successful AI model is a robust dataset. High-quality data ensures that the AI system can learn meaningful patterns and make accurate predictions. Conversely, if an AI model is trained on poor-quality data, it will inevitably produce unreliable results. This concept is often summarized by the phrase “garbage in, garbage out” (GIGO).
Case Study: The Perils of Poor Data Quality
Consider a hypothetical scenario where a financial institution uses an AI system to predict stock market trends. If the dataset used to train this AI contains outdated or incorrect information, the predictions made by the AI will be flawed. Investors relying on these predictions may make poor investment decisions, leading to significant financial losses.
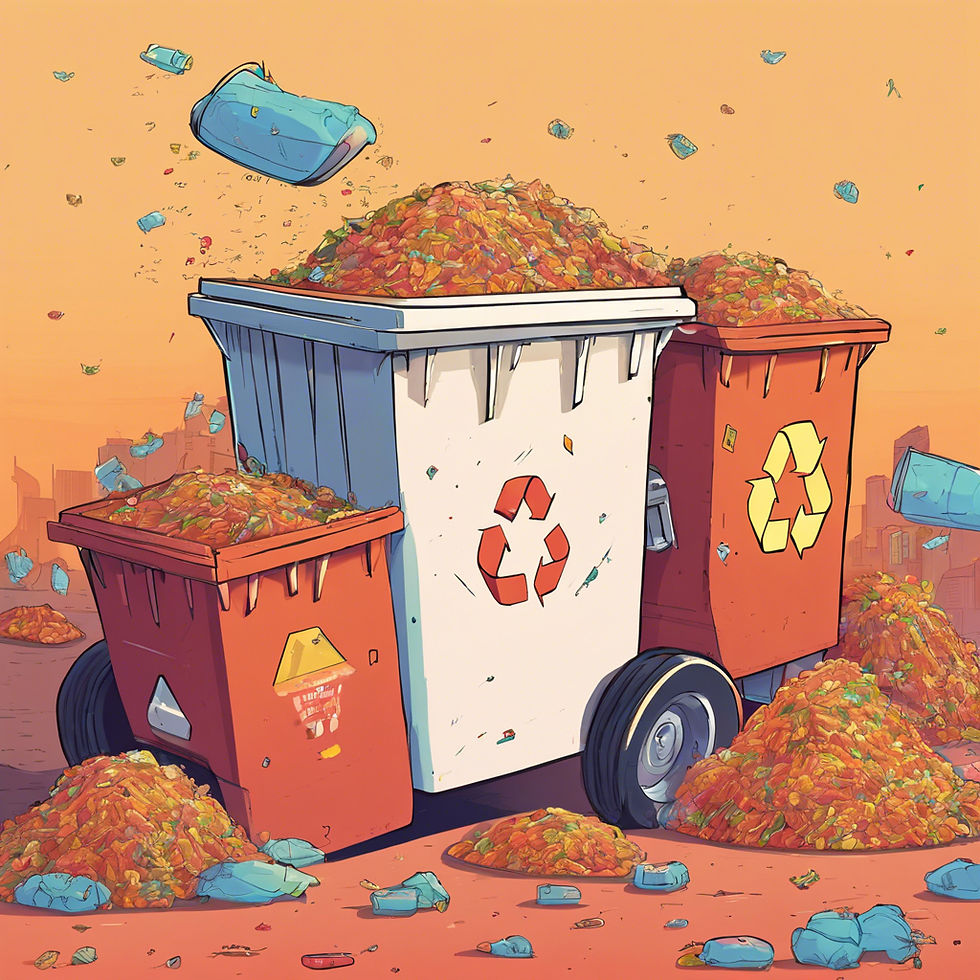
The Impact of Biased Data
Another critical issue is the presence of bias in training data. Bias can manifest in various forms, such as racial, gender, or socioeconomic biases. When AI systems are trained on biased data, they can perpetuate and even amplify these biases, leading to discriminatory outcomes.
Real-World Example: Bias in Criminal Justice AI
In the criminal justice system, AI tools are increasingly used to assess the risk of reoffending. However, if the data used to train these tools is biased against certain demographic groups, the AI may unfairly label individuals from these groups as high-risk. This can result in harsher sentencing and perpetuate systemic inequalities.
Addressing the Data Quality Challenge
To mitigate the risks associated with low-quality and biased data, it is essential to implement rigorous data collection and validation processes. Here are some key strategies:
Data Cleaning and Preprocessing: Ensure that the data is free from errors, inconsistencies, and duplicates. This step involves removing irrelevant information and standardizing the data format.
Bias Detection and Mitigation: Use techniques such as fairness-aware machine learning to identify and reduce biases in the dataset. This can include reweighting data samples or using adversarial debiasing methods.
Continuous Monitoring and Updating: AI models should be continuously monitored to detect any degradation in performance over time. Regular updates to the training data can help maintain the model’s accuracy and relevance.
Transparency and Accountability: Encourage transparency in AI development by documenting data sources, preprocessing steps, and model evaluation metrics. This helps build trust and enables independent audits.
The Role of Human Oversight
Despite advancements in AI, human oversight remains crucial. Human experts can provide valuable insights and identify potential issues that automated systems might overlook. By combining the strengths of AI and human intelligence, we can achieve more reliable and ethical AI outcomes.
Conclusion
As AI continues to evolve and integrate into various aspects of our lives, the quality of the data that feeds these systems becomes increasingly important. Ensuring high-quality, unbiased data is not just a technical challenge but a moral imperative. By addressing data quality issues, we can harness the true potential of AI while minimizing the risks of misinformation and discrimination.
The journey towards responsible AI development is ongoing, and it requires collaboration, vigilance, and a commitment to ethical principles. Only then can we create AI systems that truly benefit society and drive positive change.
Let's build a Secure future where humans and AI work together to achieve extraordinary things!
Let's keep the conversation going!
What are your thoughts on the limitations of AI for struggling companies? Share your experiences and ideas for successful AI adoption.
Contact us(info@drpinnacle.com) today to learn more about how we can help you.
Author: Vishwa Akuthota
Founder of Dr. Pinnacle and Chief AI Officer
Passionate about responsible AI and its impact on society
Comments